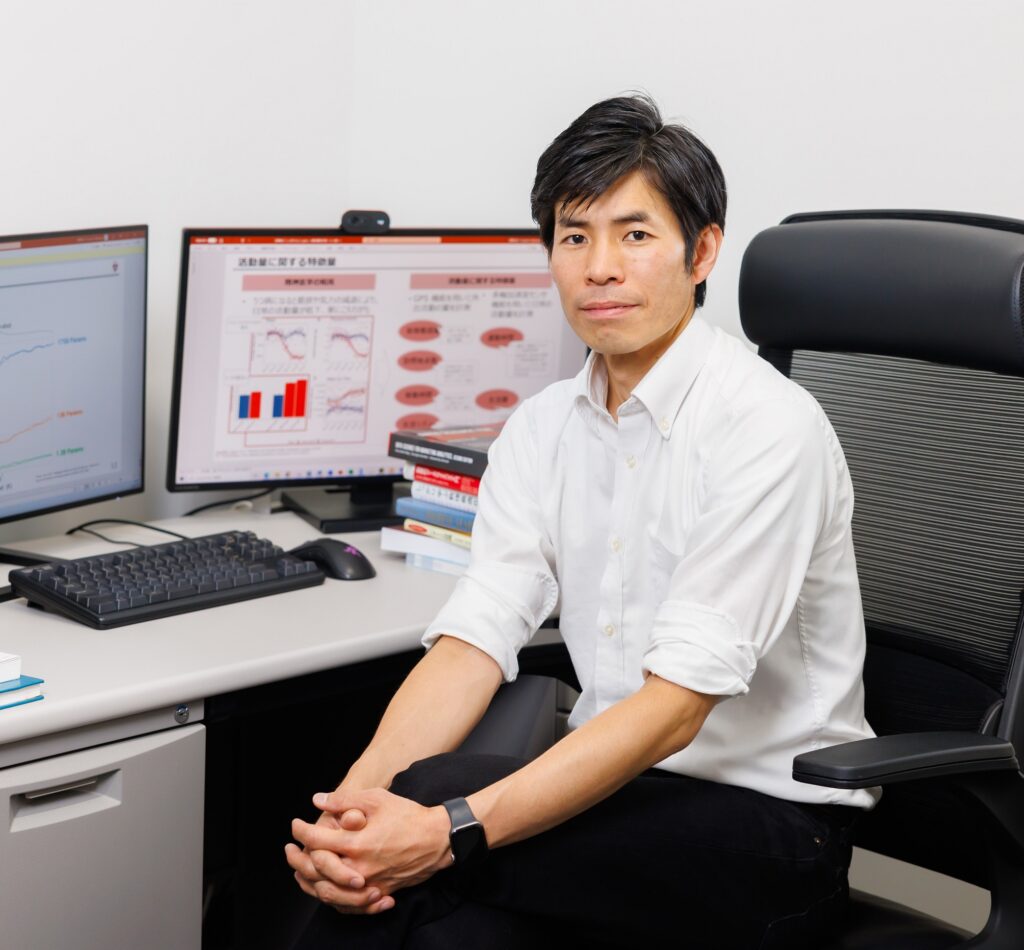
Associate professor Yusuke Fukazawa from the Graduate Degree Program of Applied Data Science undertakes research on understanding human’s mental state and behavior using data derived from smartphones and social media. Here he shares research on using smartphone data to detect mental health problems, one of his many research themes.
Mental health has become one of society’s concerns. My research seeks to create a world where individuals can assess their own mental state using big data generated by smartphones and social media.
Using smartphone logs to understand movement and physical activity
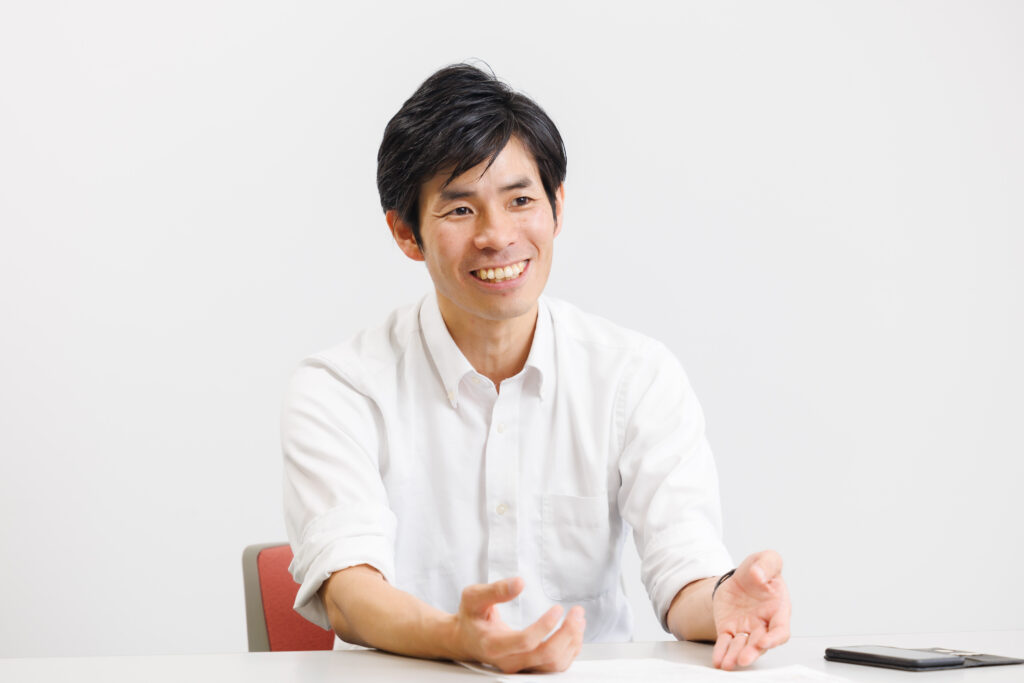
Unlike physical ailments, mental problems are often invisible. Even if they feel abnormal, they tend to pass it off as “just a little tired”.Unfortunately, attempting to push through without addressing these symptoms can result in the development of mental disorders such as depression.
I first thought of using smartphones while considering methods for identifying early-stage mental health disorders. In today’s world, where many people own and take smartphones with them wherever they go, these devices store a wide variety of data about their users. I believed this data could be used effectively.
Data from features such as the GPS function, and usage logs generated by the accelerometer (which detects movement and tilt) and the brightness sensor (which adjust the screen’s brightness according to the illumination of the surroundings) are relevant to the research.
GPS location information enables the calculation of the distance traveled by a user, while the accelerometer data provides insights into the users’ movements and physical activities based on step count and walking speed.
Additionally, screen brightness and screen-lock data reveal periods of inactivity when the devices is not in use. When we combine the smartphone’s brightness and movement data with the screen’s on-off log, we can summarize whether or not the smartphone being used in the dark.
Mental health state can be inferred with 70% to 80% accuracy
With the help of psychiatrists, I also conducted experiments to see this data accurately reflects a person’s mental state. In the experiments, participants provided consent to obtain the necessary usage logs from their smartphones, and fitted wearable devices to capture heart rate data as an indicator for mental stress.
All data obtained was analyzed using machine learning. As a result of repeating similar experiments while adjusting the combination of smartphone data, it became possible to infer mental health state to an accuracy of 70% to 80%.
Besides mental health, the data generated by people through the use of smartphones, social media, and such also has the potential for use in various fields. If we can predict people’s movements in the real world, we will be able to develop solutions for issues in traffic and transportation. We can also provide even more personalized services for each individual if we understand the purpose of people’s actions.
In the future, with the latest artificial intelligence (AI) technologies as the theoretical foundation, I hope to take on the challenge of various research themes that can help to improve the people’s quality of life through integrated processing and analysis of a broad and diverse range of human behavioral data generated by various human activities.
The book I recommend
“Taikyokukan”(Seeing the Big Picture)
by Yoshiharu Habu, Kadokawa Shinsho
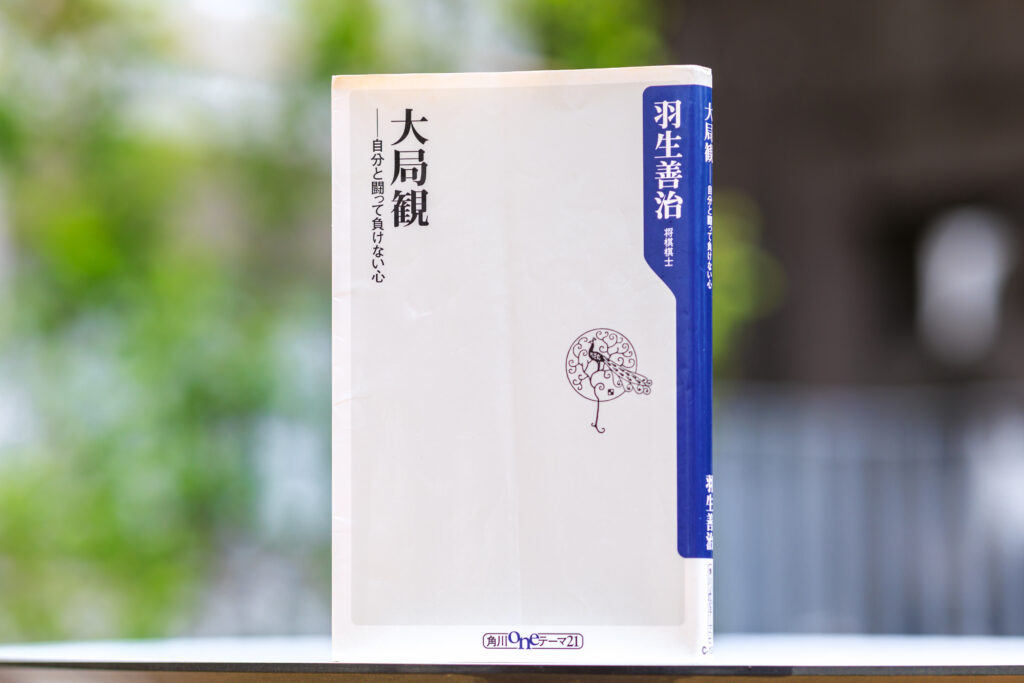
Shogi player Yoshiharu Habu shares how the practical experience gained from playing many matches helps foster his ability to solve unfamiliar problems. He calls this approach taikyokukan (seeing the big picture). While recognizing the importance of firsthand experiences, I am curious about the potential of AI to replicate this ability and its implications.
-
Yusuke Fukazawa
- Associate Professor
Graduate Degree Program of Applied Data Science
- Associate Professor
-
Graduated from the Faculty of Engineering, the University of Tokyo, and received his M.Eng. and Ph.D. at the university’s Graduate School of Engineering. Worked at NTT DOCOMO, INC. before assuming current position in 2023. Was a visiting researcher at Research into Artifacts, Center for Engineering (RACE). Currently also working as an invited researcher at Waseda Innovation Laboratory, Waseda University.
- Graduate Degree Program of Applied Data Sciences
Interviewed: May 2023